Machine Learning and Geothermal Energy
Machine learning automates a mathematical model from given data and makes predictions or decisions.
CHALLENGE
Advancement was needed in geothermal exploration to make it more reliable and predictable. The Department of Energy believed applying machine learning techniques to geothermal exploration and production could assist in finding and developing new geothermal resources.
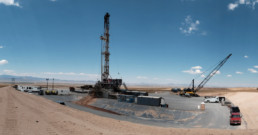
APPROACH
Machine Learning offers substantial opportunities for technology advancement and cost reduction throughout the geothermal project lifecycle, from resource exploration to power plant operations. It can help decide where drilling for geothermal well should take place and predict whether a site geologists pick contains high temperature geothermal resources.
The Utah FORGE (Frontier Observatory for Research in Geothermal Energy) site was chosen by the Department of Energy (DOE) as the winning location for testing and demonstrating new technologies that will advance geothermal heat extraction from naturally fractured, low-permeability host rocks—in particular, enhanced geothermal systems (EGS) energy development.
The Utah Geological Survey compiled geothermal information from a variety of publicly available sources including the Southern Methodist University Geothermal Laboratory, U.S. Geological Survey, recently released industry data, and internal unpublished reports. The data consist of 979 records for 952 boreholes throughout Utah.
RESULTS
Using the data supplied from the Utah Geological Survey, the wells were graded and the data labeled. An algorithm was created using Support Vector Machines or SVMs (SVMs are supervised learning models that are widely used for data classification). The algorithm was used to build a model that could make geothermal well predictions.
During subsequent tests and actual drilling the prediction the algorithm made were accurate. Subsequent testing, in other countries, proved the SVM models returned a high prediction accuracy.